In the quest to deepen our understanding of the North Atlantic Oscillation (NAO)—a climatic phenomenon that significantly influences weather patterns across Europe and North America—scientists have turned to innovative analytical methods.
A recent study leverages the power of Autoencoders (AE), a type of artificial neural network, to analyze Sea Level Pressure (SLP) data, unveiling patterns that traditional methods might overlook. This approach contrasts with the conventional Empirical Orthogonal Function (EOF) method, which has been the standard for identifying the primary modes of climate variability.
QUICK BACKGROUND
The North Atlantic Oscillation (NAO) is a unitless parameter that many meteorologists track that calculates a value based on the placement of different puzzle pieces of the atmosphere. It can significantly influences winter weather patterns across the United States, particularly along the East Coast.
During its positive phase, the NAO often leads to warmer, milder winters in the northern US, while the southeastern states may experience increased rainfall and stormier conditions. In contrast, a negative NAO phase can bring colder and snowier winter weather to the eastern part of the country.
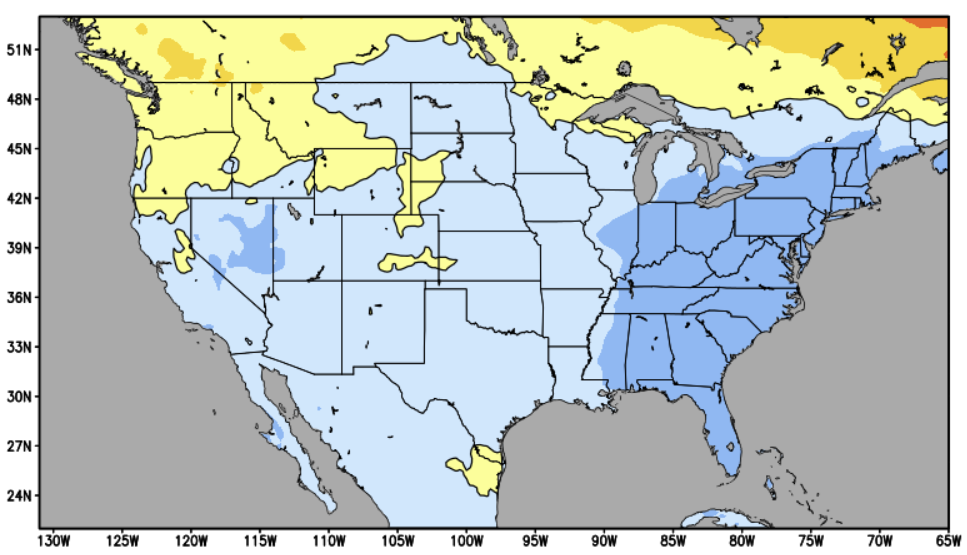
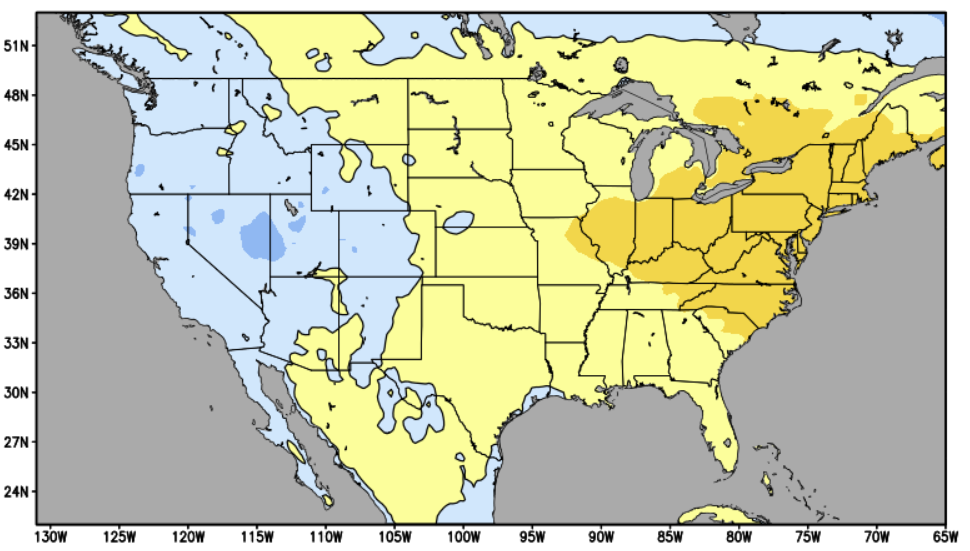
The NAO can also impact the weather along the Gulf Coast of the United States, albeit more indirectly compared to its effects on the Eastern Seaboard. During a positive phase of the NAO, the Gulf Coast may experience milder and somewhat wetter conditions, as the prevailing weather patterns can lead to increased moisture being pulled from the Gulf of Mexico. This may also, indirectly, lead to a more active severe weather scenario.
Conversely, during a negative NAO phase, the region might see slightly cooler and drier conditions, especially in the winter months, due to a shift in the jet stream that influences the movement of weather systems across the continent. While the NAO’s influence is more pronounced in the eastern and northern parts of the US, its phases can still subtly affect temperature and precipitation patterns along the Gulf Coast, contributing to the variability of winter weather experienced in the region.
Understanding the NAO’s current phase can thus provide valuable insights into seasonal weather forecasts, helping predict extreme weather events and temperature variations.
RESULTS
The findings from this cutting-edge research are revealing. By comparing the traditional EOF method with the novel AE approach, scientists have discovered that Autoencoders provide a more nuanced view of the NAO’s spatial patterns.
Notably, the AE method identified several dipole SLP patterns in the North Atlantic—complex configurations characterized by opposing centers of action—that extend beyond the classic NAO pattern. These patterns, which include variations like the Atlantic Meridional mode and the Atlantic tripole mode, suggest a rich tapestry of climatic interactions previously undocumented.
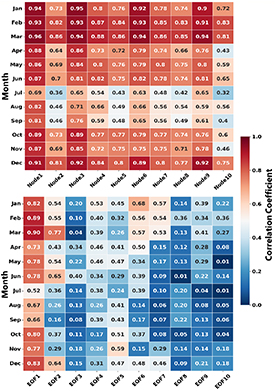
The study’s analysis showed that the AE-based patterns are closely aligned with the NAO’s spatial structure, exhibiting significant correlations with other key climatic indices such as the Arctic Oscillation (which was mostly known through previous research) and the Atlantic Meridional Mode.
This highlights the intertwined nature of climatic modes in the North Atlantic region. Furthermore, the AE approach outperformed the EOF method in capturing the essence of the NAO, especially during the boreal winter months when the NAO exerts its most substantial influence. The AE-based method not only revealed non-linear patterns of the NAO but also demonstrated a higher correlation with the station-based NAO index, providing a more comprehensive representation of this climatic phenomenon.
The results should help climate science and weather prediction. By employing Autoencoders to analyze climatic data, researchers can unlock a deeper comprehension of the NAO and its complex interactions with other climate modes. This advanced analytical capability could lead to significant improvements in seasonal weather forecasts, particularly in regions heavily influenced by the NAO, such as Europe and the eastern United States.
The success of the AE method in this study also paves the way for applying machine learning techniques more broadly in climate research, offering promising avenues for future investigations and enhancing our ability to model and respond to complex climate phenomena.
In summary, this groundbreaking research marks a significant step forward in our quest to understand the intricate dynamics of the North Atlantic Oscillation and its impact on global weather patterns. By harnessing the power of artificial neural networks, scientists are opening new frontiers in climate science, promising more accurate predictions and a better understanding of the Earth’s climatic system.
To read more bout this new research you can find it here: https://www.climate.gov/news-features/feed/machine-learning-method-leads-better-understanding-north-atlantic-oscillation?fbclid=IwAR0UMvb5TOCXQFaVjAIZNqnFUgYqQY8P2Gnd6231VNZP0qrRxCjVshDY3VA